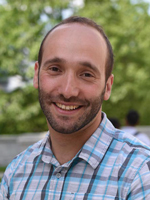
Michael Baym
Harvard Medical School - DBMI
Countway 303
695 Huntington Ave.
Boston, MA 02115
Email: baym@hms.harvard.edu
Website: baymlab.hms.harvard.edu
Research Summary
Antibiotic resistance is a quintessential systems problem. While in certain cases it can result from a single mutation in a single gene, the magnitude and effect of resistance can be dynamic and context-dependent – it can be difficult or impossible to predict from simple tests and a classical, reductionist understanding. My lab seeks to understand the basic science of the evolution and spread of antibiotic resistance, while remaining grounded and guided by the applied problem of fighting the growing public health threat.
With a combination of experimental evolution, algorithm development, and big-data analysis, we endeavor to enable a future in which we “play one move ahead” of antibiotic resistance by manipulating evolution to induce antibiotic sensitivity.
Publications
-M. Baym, T.D. Lieberman, E.D. Kelsic, R. Chait, R. Gross, I. Yelin, R. Kishony, Spatiotemporal microbial evolution on antibiotic landscapes, Science, 353 1147-1151 (2016)
-Y. J. Jiao*, M. Baym*, A. Veres, R. Kishony, Population diversity can jeopardize the efficacy of antibiotic cycling, in review
Preprint: http://www.biorxiv.org/content/early/2016/10/20/082107
These two papers, when taken together with the ideas presented in a review article I wrote with Roy Kishony last year, lay the foundation for the research program above. Through both adding a spatial dimension to experimental evolution (Baym, et al), and increasing the scale of experiments (Jiao, et al), we show how context-dependent and rare mutations can affect the course of resistance evolution and confound efforts to control it. Indeed, the heterogeneous nature of cross-resistance to antibiotics between different resistance mutations to the same antibiotic implies that evolutionarily-guided treatment strategies will have to be responsive to the particularities of the resistance phenotype and its context. Indeed, this shows that a reductionist, single-mutation single-effect model of resistance is insufficient, and that a systems understanding is required to effectively address the problem.
-P.-R. Loh*, M. Baym*†, and B. Berger†, Compressive Genomics, Nature Biotechnology 30 627–630 (2012)