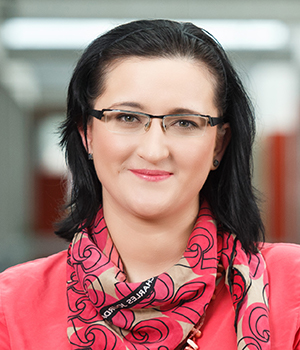
Marinka Zitnik
Countway Building, Room 309
Department of Biomedical Informatics
10 Shattuck Street
Boston, MA 02115
Tel.: 617-432-5138 and 650-308-6763
Email: marinka@hms.harvard.edu
Website: zitniklab.hms.harvard.edu
Admin: Kristen O’Connor, Kristen_OConnor@hms.harvard.edu
Research Summary
Networks pervade biomedical systems—from the molecular level to the level of dependencies between diseases in a person and all the way to the population level encompassing all human interactions. These interactions at different levels give rise to a bewildering degree of complexity that is only likely to be fully understood through a holistic and integrated systems view. My lab seeks to understand key mechanisms of networked systems and infuse them into machine learning solutions that can optimize and manipulate the systems and predict their behavior.
Our research realizes a scientific approach in which we invent ways to combine rich, heterogeneous data in their broadest sense to reduce redundancy and uncertainty and to make them amenable to comprehensive analyses. Using these rich data representations, we develop machine learning methods for learning actionable representations and translate machine learning research into solutions for open biomedical questions. Our research proves that this approach not only opens up new avenues for understanding nature, analyzing health, and developing new medicines to help people but can impact the way predictive modeling is performed today at the fundamental level.
Our current research directions include: fusing data modalities like genetic code, behavior, therapeutics, nutrients, and the environment into knowledge networks; pioneering graph neural networks and deep learning for networks to reason about interconnected biology and medicine; developing methods for learning representations that are actionable—lend themselves to actionable hypotheses—and allow users of our models to ask what-if questions and receive predictions that are accurate, precise, robust and can be interpreted meaningfully; advancing algorithms to train more with less data, exploit the ability of models to apply prediction prowess acquired from one data type to another type, and design contextually adaptive AI for classes of phenomena that can learn about never-before-seen phenomena (e.g., new patients, diseases, or cell types); and developing AI infrastructure for discovery of safe and effective therapeutics.
Publications
M. Zitnik, R. Sosic, M.W. Feldman, J. Leskovec. Evolution of Resilience in Protein Interactomes Across the Tree of Life. Proceedings of the National Academy of Sciences (PNAS), 116, 10:4426-4433, 2019.
E. Alsentzer, S.G. Finlayson, M.M. Li, M. Zitnik. Subgraph Neural Networks. Proceedings of Neural Information Processing Systems, NeurIPS, 34, 2020.
M. Zitnik, R. Sosic, J. Leskovec. Prioritizing Network Communities. Nature Communications, 9, 2544, 2018.