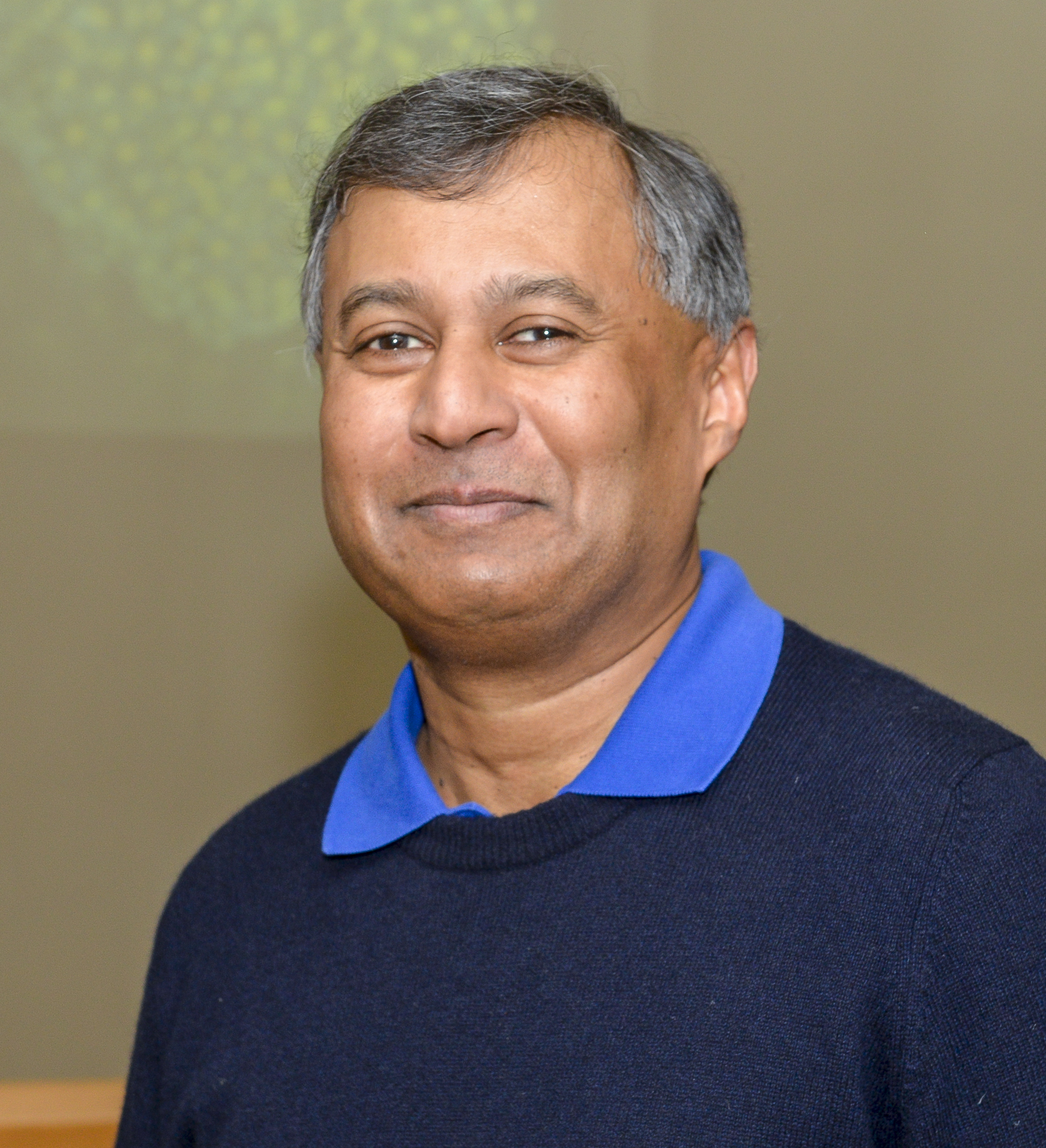
Jeremy Gunawardena
Harvard Medical School, Department of Systems Biology
Goldenson Building, Room 509
200 Longwood Ave.
Boston, MA 02115
Tel: 617-432-4839
Email: jeremy_gunawardena@hms.harvard.edu
Website:
http://vcp.med.harvard.edu/index.html
Lab Size: Between 5 and 10
Summary
How do mammalian cells process the information they receive from chemical signals and decide what to do? We take two broad approaches to studying this. From the “inside out”, we study particular molecular mechanisms which are implicated in cellular information processing, such as protein post-translational modification (PTM). Most cellular proteins are post-translationally modified in multiple ways on multiple sites, giving an enormous range of combinatorial molecular states and, with it, the potential capability for sophisticated information “encoding” [4]. We have developed biophysical methods (mass spectrometry and nuclear magnetic resonance spectroscopy) for accurately measuring PTM states, at least for proteins with small (< 10) numbers of sites, [6]. We use this in combination with “systems biochemistry” to try and undertstand how networks of enzymes regulate the distribution of PTM states and thereby control cellular behaviour.
The combinatorial complexity in PTM is a particular challenge to our conceptual understanding [4]. We have pioneered two mathematical strategies for overcoming this. We have developed a “linear framework” for undertaking time-scale separation, which can analytically simplify extremely complex biochemical networks [5,7,8]. We are now exploiting this framework to study gene regulation in eukaryotic genomes, where the framework allows us to accommodate dissipative, non-equilibrium mechanisms like histone modifications and nucleosome reorganisation. We have also developed the method of “invariants”, in which we exploit the fact that, because of mass-action, a network of (bio)chemical reactions gives rise to a polynomial dynamical system. We can therefore use methods from algebraic geometry to analyse such networks at steady state [2,3,10]. For instance, we can determine (at least in principle) the algebraic invariant that holds among specified components in a network, while eliminating the influence of all the remaining components [3,10]. This distillation can make it much easier to understand what the network is doing. Among other things, these new mathematical developments give us the capability to rigorously infer the properties of certain systems, including PTM systems, irrespective of their underlying molecular complexity [2,8]. We are seeing in this way the emergence of new kinds of mathematics for overcoming biological complexity.
Our second broad approach is from the “outside in”. This arises from a different perspective, which suggests that the complexity found in biochemical networks cannot be fully explained by just studying the networks. Instead, this internal complexity reflects the external complexity of the environments in which these networks were evolved. We have developed new types of programmable microfluidic device to subject cells to complex “interrogation”. We use fluorescence microscopy to assay downstream behaviours at single-cell level and use mathematical models of the molecular networks to predict what we should see. We have developed flexible computational infrastructures (“little b” and, more recently, “Proteus”) to support this kind of modelling, [9]. Instead of studying networks by pulling them to pieces, as in our first approach, we try to ask cells more complicated questions, in the hope that their answers will reveal more about how they work.
One of the most awkward problems in studying signalling is that each cell is an individual entity and may respond to a signal differently from its neighbours, even in a clonal population. This cell-to-cell variation is obscured by population averages like Western blots. We try and use the variation to glean more information about the networks but cell culture is such an artificial environment that the variation is hard to interpret biologically. We have now started exploring more physiological contexts, including embryonic stem cells.
Systems biology is a fascinating area in which ideas from mathematics, physics and engineering are starting to provide new conceptual insights into biology. There are no shortage of hard questions for those who enjoy prospecting in unexplored territory.
Publications
N Hao, B A Budnik, J Gunawardena, E K O'Shea, "Tunable signal processing through modular control of transcription factor translocation", Science, 339:460-4 2013.
Y Xu, J Gunawardena, "Realistic enzymology for post-translational modification: zero-order ultrasensitivity revisited", J Theor Biol, 311:139-52 2012.
R L Karp*, M Peréz Millán*, T Dasgupta*, A Dickenstein, J Gunawardena, "Complex-linear invariants of biochemical reaction networks", J Theor Biol, 311:130-8 2012.
S Prabakaran, G Lippens, H Steen, J Gunawardena, "Post-translational modification: nature's escape from genetic imprisonment and the basis for dynamic information encoding", WIREs Systems Biology and Medicine, 4:565-83 2012.
J Gunawardena, "A linear framework for time-scale separation in nonlinear biochemical systems", PLoS ONE, 7:e36321 2012.