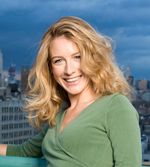
Franziska Michor
The Center for Life Sciences Building
3 Blackfan Circle
Boston, MA 02115
Email: michor@jimmy.harvard.edu
Website: http://michorlab.dfci.harvard.edu/
Lab size: between 15 and 20
Summary
We are interested in using the tools of theoretical evolutionary biology, applied mathematics, statistics, and computational biology to address important questions in cancer research.
Current projects include the following:
(1) Which genetic and epigenetic alterations cause cancer?
Cancer cells often harbor hundreds to thousands of genetic changes. Many of those changes represent neutral variation that does not influence cancer development; such mutations are called passenger or hitchhiking mutations. A few alterations, however, are essential for driving tumorigenesis. Those changes are known as driver mutations and increase the reproductive fitness of the cancer cell. The identification of such mutations is of crucial importance for drug discovery because they represent promising targets for therapeutic intervention. We are developing a novel evolutionary mathematical framework designed to identify alterations that act as drivers during tumorigenesis. This method may aid in the prioritization of candidate mutations for functional validation and contribute to the process of drug discovery.
(2) In which order do oncogenic alterations arise?
Recent technological advances have empowered researchers to examine the cancer genome at unprecedented throughput and resolution. Computational algorithms designed to filter random genetic events have begun to uncover mutational patterns that are typical for a particular cancer type and highly consistent between sample sets. Further functional validation of these recurrent genetic events in non-transformed primary cells and mouse models of human cancer is hampered by the lack of knowledge of the sequence in which these alterations occur during human tumorigenesis. This temporal order can guide the generation of the correct genomic context in animal models of human cancer and can prioritize the validation of potential drug targets since those changes that occur early during malignant transformation may result in rewiring of the signaling circuitry or confer a state of addiction to the new signal. We have designed a novel computational approach, calledRetracing the Evolutionary Steps In Cancer (RESIC), to determine the sequence of genetic events using cross-sectional genomic data from a large number of tumors at their fully transformed stage. RESIC represents the first evolutionary mathematical approach to identify the temporal sequence of mutations driving tumorigenesis and may be useful to guide the validation of candidate genes emerging from cancer genome surveys.
(3) Where do oncogenic alterations arise?
Two independent theories for the initiation of human cancers are often presented: a) the “stem cell” theory and b) a theory that espouses “dedifferentiation of more differentiated cells and their acquisition of self-renewal”. The degree to which each of these theories represents the actual events occurring during human tumorigenesis is unclear, and due to the diverse nature of cancer, it is possible that each model may hold true in different circumstances. We are currently investigating this issue in two cancer types: in the case of myeloid malignancies, including acute and chronic leukemias, there is evidence to suggest that hematopoietic stem cells are the cell of origin, whereas in other cases the malignant clone may emerge from a more differentiated component of the hematopoietic compartment. However, unlike for B-cell neoplasms, where immunoglobulin rearrangements can be used to lineage trace the malignant clone, there are no definitive data from genetic studies of human cells or from murine models which elucidate the cell of origin for acute and chronic myeloid leukemias. In the second case of glioma, some data suggests that the cell of origin is a stem cell or at least resides in the stem cell niche (the subventricular zone) while other data suggests that cells of various regions of the brain or differentiation status could serve as the cell of origin, especially if the oncogenes inducing the tumor undermine the ability for the cell of origin to maintain a differentiated state. We are using evolutionary mathematical modeling to identify the cell of origin of human cancers and, in collaboration with the Levine and Holland labs at Memorial Sloan-Kettering Cancer Center, test the predictions in experimental mouse models of the disease.
(4) How do oncogenic alterations arise?
Several large-scale cancer genome profiling studies have recently been performed, which have identified loci that are altered in many cancer samples. Although many of those loci harbor known oncogenes and tumor suppressor genes, some regions span tens of kilobases containing multiple genes or gene desert regions. Interestingly, DNA breakpoints in cancer genomes and also in the genomes of apparently healthy human subjects are distributed non-randomly, suggesting that some regions within the human genome, so-called breakpoint hotspots, can be exquisitely prone to rearrangement of genetic material. Many such regions are specific to particular cancer types, indicating that genomic instability may manifest itself differentially in neoplasms of diverse origin. While in some cases, exogenous factors may cause mutations, in other cases endogenous factors may play a more important role. For instance, epigenetic signatures such as DNA methylation patterns differ between cell types and are known to be associated with genomic instability during both the somatic evolution of cancer and germline evolution during speciation. However, the mechanisms underlying the generation of genomic rearrangements and their relation to methylation patterns are still incompletely understood. To elucidate potential endogenous mechanisms of cancer genome evolution, we have embarked on a comprehensive computational investigation of DNA amplification and deletion data, methylation information, and structural predictions that has already led to some mechanistic hypotheses about the generation of DNA breakpoints in cancer.
(5) What do we do about it?
- Dynamics of treatment response
Targeted anti-cancer therapy promises efficient and safe treatment by interfering with a specific molecular target that has a crucial role in tumor growth or progression. The clinical success of the small-molecule kinase inhibitor imatinib mesylate (Gleevec, STI571) in treating chronic myeloid leukemia (CML) sets the standard for such therapy. Imatinib causes a rapid depletion of the leukemic cell burden and leads to remissions in all stages of the disease. In most patients, however, imatinib fails to eliminate residual disease. Furthermore, acquired resistance to imatinib is an emerging problem in the treatment of CML. The ability to determine the disease burden by quantitative PCR of the BCR–ABL oncogene (a fusion gene comprising breakpoint cluster region and Abelson murine leukemia viral oncogene homolog 1), which drives CML, facilitates the development of quantitative approaches to investigate these issues. The goal of such studies is to explain some key features of the data: (i) imatinib therapy leads to a biphasic decline of the leukemic cell burden during the first two years of therapy; (ii) discontinuation of imatinib therapy causes a rapid resurgence of the leukemic cell load in some patients; and (iii) evolution of resistance leads to an increase in the number of leukemic cells, despite continuous therapy. We are developing mathematical and statistical models to investigate the dynamics of treatment response in patients with CML, multiple myeloma, prostate cancer, brain cancer, breast cancer, and other diseases.
- Classification of tumors for prognostic purposes
Gene expression profiling of malignancies is often held to demonstrate genes that are ‘‘up-regulated’’ or ‘‘downregulated’’, but the appropriate frame of reference against which observations should be compared has not been determined. Fully differentiated somatic cells arise from stem cells, with changes in gene expression that can be experimentally determined. If cancers arise as the result of an abruption of the differentiation process, then poorly differentiated cancers would have a gene expression more similar to stem cells than to normal differentiated tissue, and well differentiated cancers would have a gene expression more similar to fully differentiated cells than to stem cells. We have developed a novel computational algorithm that allows orientation of cancer gene expression between the poles of the gene expression of stem cells and of fully differentiated tissue. Our methodology allows the construction of a multi-branched phylogeny of human malignancies and can be used to identify genes related to differentiation as well as novel therapeutic targets. Our algorithm represents the first phylogeny-based tool to analyze the differentiation status of human tumors, and has proven predictive power for survival of ovarian, breast, colon, and hematologic cancer patients. We are currently investigating similar methods for other cancer and data types.
- Identification of optimum administration schedules of anti-cancer therapies
Acquired resistance to anti-cancer therapy is a major limitation of existing treatment modalities. A novel way to examine the problem of acquired resistance is to look at it as a problem in evolution. As such, mathematical modeling is ideal to model evolutionary processes, and such modeling can provide new insights into the biology of cancer. We are using mathematical modeling to address several paradigmatic clinic scenarios. Using multi-type non-homogeneous birth–death processes as well as pre-clinical human lung cancer data, we have identified several treatment schedules of targeted agents that are predicted to perform superior to currently used clinical strategies; these are now in clinical trials in Asia and the US. Using dynamical systems modeling, we have identified better radiation treatment schedules for brain cancer that will be implemented as clinical trials hopefully next year. Using agent-based stochastic simulation modeling of patient-specific scenarios, we have also studied the effects of neoadjuvant treatment in breast cancer and have predicted better intervention schedules. We are extending this type of investigation to other cancer and treatment types.